BUF-Unet
dental image segmentation by introducing BEF-Unet
Within the field of oral healthcare, precise teeth segmentation is a critical component, providing essential positional data for orthodontic procedures, clinical diagnoses, and surgical interventions. While convolutional neural networks (CNNs) have been widely employed for image segmentation, they face limitations in capturing a comprehensive global context and long-range relationships, particularly when dealing with the diverse textures, scales, and shapes found in medical images. On the other hand, vision transformers show promise in grasping broader contextual information but struggle with the spatial intricacies of image data. To address these challenges, the DenUnet architecture is proposed, combining the strengths of CNNs and transformers. It features a dual-branch encoder for simultaneous edge and body information extraction, a DoubleLevel Fusion module for multi-scale feature integration, and a Local Cross-Attention Feature fusion module to enhance feature fusion. Notably, the architecture incorporates a novel multi-scale vision transformer. Experimental results highlight DenUnet’s superior effectiveness in dental image segmentation, particularly in handling irregular boundaries. The key contributions of this work are the innovative fusion of edge and body information, the enhancement of feature representations through the Double-Level Fusion module, and the robust performance of DenUnet across dental image datasets.
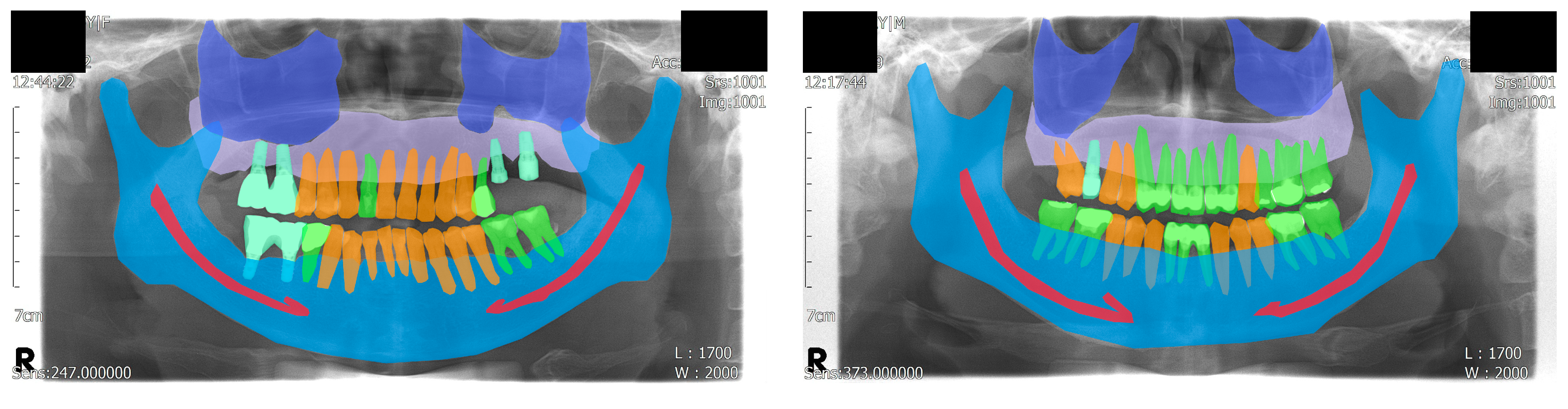